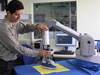
Kormushev, P., Calinon, S. and Caldwell, D.G. (2010)
Approaches for Learning Human-like Motor Skills which Require Variable Stiffness During Execution
In Proc. of the IEEE Intl Conf. on Humanoid Robots (Humanoids), Workshop on Humanoid Robots Learning from Human Interaction, Nashville, TN, USA.
Abstract
Humans employ varying stiffness in everyday life for almost all human motor skills, using both passive and active compliance. Robots have only recently acquired variable passive stiffness actuators and they are not yet mature. Active compliance controllers have existed for a longer time, but the problem of automatic determination of the necessary compliance to achieve a task has not been thoroughly studied. Teaching humanoid robots to apply variable stiffness to the skills they acquire is vital in order to achieve human-like naturalness of the execution. Also, using adaptive compliance can help to increase the energy efficiency. This paper compares two different approaches that allow robots to learn human-like skills which require varying stiffness during execution. The advantages and disadvantages of each approach is discussed and demonstrated with various experiments on an activelycompliant Barrett WAM robot.
Bibtex reference
@inproceedings{Kormushev10ws, author="Kormushev, P. and Calinon, S. and Caldwell, D. G.", title="Approaches for Learning Human-like Motor Skills which Require Variable Stiffness During Execution", booktitle = "{IEEE} Intl Conf. on Humanoid Robots ({H}umanoids), Workshop on Humanoid Robots Learning from Human Interaction", month = "December", year = "2010", address = "Nashville, TN, USA", }
Video
The video shows a Barrett WAM 7 DOFs manipulator learning to flip pancakes by reinforcement learning. The motion is encoded in a mixture of basis force fields through an extension of Dynamic Movement Primitives (DMP) that represents the synergies across the different variables through stiffness matrices. An Inverse Dynamics controller with variable stiffness is used for reproduction.
The skill is first demonstrated via kinesthetic teaching, and then refined by Policy learning by Weighting Exploration with the Returns (PoWER) algorithm. After 50 trials, the robot learns that the first part of the task requires a stiff behavior to throw the pancake in the air, while the second part requires the hand to be compliant in order to catch the pancake without having it bounced off the pan.
Video credits: Dr Petar Kormushev, Dr Sylvain Calinon