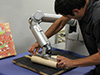
Calinon, S., Alizadeh, T. and Caldwell, D.G. (2013)
On improving the extrapolation capability of task-parameterized movement models
In Proc. of the IEEE/RSJ Intl Conf. on Intelligent Robots and Systems (IROS), Tokyo, Japan, pp. 610-616.
Abstract
The movement generalization problem in robot learning by imitation is challenging, because of the small number of demonstrations provided by the user, and because of the varying accuracy and correlation requirements. Movements are most often driven by landmarks in task space (real or virtual) that can change during the course of the movement and that requires diverse types of constraints. We present an approach to statistically encode series of movements in a task-parameterized model, and derive an expectation-maximization (EM) algorithm to train it. The model automatically extracts the relevance of candidate coordinate systems during the task, and exploits this information during reproduction to adapt the movement in real-time to changing position and orientation of landmarks or objects. The approach is tested with a robotic arm learning to roll out a pizza dough. It is compared to three categories of task-parameterized models: 1) Gaussian process regression (GPR) with a trajectory models database; 2) Multi-streams approach with models trained in several frames of reference; 3) Parametric Gaussian mixture model (PGMM) modulating the Gaussian centers with the task parameters. We show that the extrapolation capability of the proposed approach go beyond existing methods, by extracting the structure of the task in the form of local transformations, instead of relying on interpolation principles.
Bibtex reference
@inproceedings{Calinon13IROS, author="Calinon, S. and Alizadeh, T. and Caldwell, D. G.", title="On improving the extrapolation capability of task-parameterized movement models", booktitle="Proc. {IEEE/RSJ} Intl Conf. on Intelligent Robots and Systems ({IROS})", year="2013", month="November", address="Tokyo, Japan", pages="610--616" }
Video
The aim of robot learning by imitation is to provide user-friendly means of transferring skills to robots, by exploiting the natural teaching capability of the users. Imitation is not simply recording and replaying movements. Instead, the learned skills require to be generalized to new situations.
Machine learning can help to extract relevant patterns from multiple demonstrations of the skill (invariant characteristics of the task). The research challenge is to develop tools with good extrapolation capability and working with small datasets. In learning from demonstrations, the robot should be able to start generalizing the task early in the interaction. This can be done in several ways, with techniques that can learn the underlying structure of the task, that can extract the meanings behind the actions, or that can understand how the robot, user and environment can modify the task.
The user can increase the robot's learning speed by providing several relevant examples of the same task. By scaffolding the environment and by introducing variability, the robot can then extract which parts of the movement matter the most, and how the movement is modulated by external cues such as positions of objects.
The more complex the task are, the more difficult it is for the user to pre-determine and keep track of the possible variations of a task. To reduce this cognitive load, it might thus be relevant to consider machine learning approaches that can not only interpolate between multiple demonstrations, but that can also extrapolate the task to new situations that could be far from the observed situations. Such extrapolation capability would avoid that the requirement of carefully covering all the possible situations in which a motion can be used.
This video presents an example in which five demonstrations of rolling out a pizza dough are provided to the robot by kinesthetic teaching. The controller of the robot compensates for the gravity to facilitate the demonstrations. The user can in this way move the robot as if it had no weight and no motor in its articulations, while the robot records proprioceptive information about the position of its arm, as well as visual information about the pizza dough from an external camera. After demonstration, the robot knows how to move the rolling pin toward the dough and how to change the amplitude and direction of the movement with respect to the current shape of the dough.
The underlying goal of this task is to locally change the rolling motion such that it becomes parallel to the smallest eigenvector of the dough shape extracted by image processing. The adaptation of the movement is not provided to the robot. Instead, the robot learns how to locally modify the trajectory of the end-effector with respect to the dough position, orientation and elongation.
Source codes
Training of a task-parameterized Gaussian mixture model (GMM) based on candidate frames of reference.
The proposed task-parameterized GMM approach relies on the linear transformation and product properties of
Gaussian distributions to derive an expectation-maximization (EM) algorithm to train the model.
The proposed approach is contrasted with an implementation of the approach proposed by Wilson and Bobick in
1999, with an implementation applied to GMM (that we will call PGMM) and following the model described in
"Parametric Hidden Markov Models for Gesture Recognition", IEEE Trans. on Pattern Analysis and Machine
Intelligence.
In contrast to the standard PGMM approach, the new approach that we propose allows the parameterization of
both the centers and covariance matrices of the Gaussians. It has been designed for targeting problems in
which the task parameters can be represented in the form of coordinate systems, which is for example the
case in robot manipulation problems.
Download
Download task-parameterized GMM Matlab sourcecode
Download task-parameterized GMM C++ (command line version) sourcecode
(see also DMP LEARNED BY GMR sourcecode)
Usage
For the Matlab version, unzip the file and run 'demo1' or 'demo2' in Matlab.
For the C++ version, unzip the file and follow the instructions in the ReadMe.txt file.
Reference
- Calinon, S., Li, Z., Alizadeh, T., Tsagarakis, N.G. and Caldwell, D.G. (2012) Statistical dynamical systems for skills acquisition in humanoids. Proc. of the IEEE Intl Conf. on Humanoid Robots (Humanoids).
Demo 1 - Simple example of task-parameterized GMM learning and comparison with standard PGMM
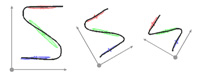
This example uses 3 trajectories demonstrated in a frame of reference that varies from one demonstration to the other. A model of 3 Gaussian components is used to encode the data in the different frames, by providing the parameters of the coordinate systems as inputs (transformation matrix A and offset vector b).
Demo 2 - Example of task-parameterized movement learning with DS-GMR (statistical dynamical systems based on Gaussian mixture regression)
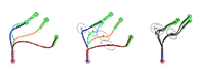
This demo shows how the approach can be combined with the DS-GMR model to learn movements modulated with respect to different frames of reference. The DS-GMR model is a statistical dynamical system approach to learn and reproduce movements with a superposition of virtual spring-damper systems retrieved by Gaussian mixture regression (GMR). For more details, see the 'DMP-learned-by-GMR-v1.0' example code downloadable from the website below.