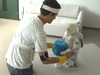
Calinon, S. and Billard, A. (2007)
What is the Teacher's Role in Robot Programming by Demonstration? - Toward Benchmarks for Improved Learning
Interaction Studies, Special Issue on Psychological Benchmarks in Human-Robot Interaction, 8:3, 441-464.
Abstract
Robot programming by demonstration (RPD) covers methods by which a robot learns new skills through human guidance. We present an interactive, multimodal RPD framework using active teaching methods that places the human teacher in the robot's learning loop. Two experiments are presented in which observational learning is first used to demonstrate a manipulation skill to a HOAP-3 humanoid robot by using motion sensors attached to the teacher's body. Then, putting the robot through the motion, the teacher incrementally refines the robot's skill by moving its arms manually, providing the appropriate scaffolds to reproduce the action. An incremental teaching scenario is proposed based on insights from various fields addressing developmental, psychological, and social issues related to teaching mechanisms in humans. Based on this analysis, different benchmarks are suggested to evaluate the setup further.
Bibtex reference
@article{Calinon07IS, author="S. Calinon and A. Billard", title="What is the Teacher's Role in Robot Programming by Demonstration? - {T}oward Benchmarks for Improved Learning", journal="Interaction Studies. {S}pecial Issue on Psychological Benchmarks in Human-Robot Interaction", editor="K. Dautenhahn", year="2007", volume="8", number="3" pages="441-464" }
Video
Incremental learning by using different teaching methods. The robot first observes the user demonstrating the skill through the use of motion sensors. Then, the user is supporting the robot during its reproduction attempts, providing scaffolds to help the robot fulfill the task constraints.
Source codes
Download
Â
Download GMR multiConstraints sourcecode
Usage
Unzip the file and run 'demo1' in Matlab.
References
- Calinon, S. and Billard, A. (2007) What is the Teacher's Role in Robot Programming by Demonstration? - Toward Benchmarks for Improved Learning. Interaction Studies. Special Issue on Psychological Benchmarks in Human-Robot Interaction. 8:3, 441-464.
Demo 1 - Demonstration of the reproduction of a generalized trajectory through Gaussian Mixture Regression (GMR), when considering two independent constraints represented separately in two Gaussian Mixture Models (GMMs)
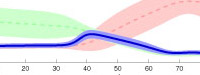
Through regression, a smooth generalized trajectory satisfying
the constraints encapsulated in both GMMs is extracted, with associated
constraints represented as covariance matrices.
The program loads two datasets, which are encoded separetely in two
GMMs. GMR is then performed separately on the two datasets, and the
resulting Gaussian distributions at each time step are multiplied to
find an optimal controller satisfying both constraints, producing a
smooth generalized trajectory across the two datasets.