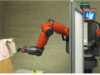
Tanwani, A.K. and Calinon, S. (2016)
Learning Robot Manipulation Tasks with Task-Parameterized Semi-Tied Hidden Semi-Markov Model
IEEE Robotics and Automation Letters (RA-L), 1:1, 235-242.
Abstract
In this paper, we investigate the use of semi-tied Gaussian mixture model for robust learning and adaptation of robot manipulation tasks. We exploit the spatial and temporal correlation in the data by tying the covariance matrices ofthe mixture model with common synergistic directions/basis vectors, instead of estimating full covariance matrices for each cluster in the mixture. This allows the reuse of the discovered synergies in different parts of the task having similar coordination patterns. We extend the approach to task-parameterized and hidden semi-Markov models for autonomous adaptation to changing environmental situations. The planned movement sequence from the model is smoothly followed with a finite horizon linear quadratic tracking controller. Experiments to encode whole body motion data in simulation, followed by valve opening and pick-and-place via obstacle avoidance tasks with the Baxter robot, show improvement over standard Gaussian mixture models with much less parameters and better generalization ability.
Bibtex reference
@article{Tanwani16RAL, author="Tanwani, A. K. and Calinon, S.", title="Learning Robot Manipulation Tasks with Task-Parameterized Semi-Tied Hidden Semi-{M}arkov Model", journal="{IEEE} Robotics and Automation Letters ({RA-L})", doi="10.1109/LRA.2016.2517825", year="2016", month="January", volume="1", number="1", pages="235--242" }
Video
In this experiment, Baxter learns to open the valve using torque control from previously unseen configurations after being demonstrated a few times only. The task is encoded with the task-parametrized semi-tied hidden semi-Markov model and the movement is reproduced with a finite horizon linear quadratic tracking controller. For more details of the proposed algorithm for learning robot manipulation tasks, please read the paper: A. K. Tanwani, S. Calinon, "Learning Robot Manipulation Tasks with Task-Parametrized Semi-Tied Hidden Semi-Markov Model", IEEE Robotics and Automation Letters, 2016.
Video credit: Ajay Tanwani
Source codes
Source codes related to this publication are available as part of PbDlib.