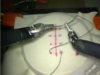
Tanwani, A.K., Yan, A., Lee, J., Calinon, S. and Goldberg, K. (2021)
Sequential Robot Imitation Learning from Observations
International Journal of Robotics Research (IJRR), 40:10-11, 1306-1325.
Abstract
This paper presents a framework to learn the sequential structure in the demonstrations for robot imitation learning. We first present a simple generative model that extracts duration segments (also called sub-goals or options) from observations, and optimally follows the sampled sequence of states from the model with a linear quadratic tracking controller. In our earlier work, we presented a task parameterized formulation of the model to adapt the model to changing environmental situations during manipulation. In this work, we present an end-to-end model for extracting sub-goals from videos that are sequenced together for performing a manipulation task. We learn a deep embedding feature space from videos by minimizing a triplet loss that pulls together different images of the same segment, while pushing away images randomly sampled from other segments. The videos are iteratively segmented for a given parametrization of the embedding space with an Expectation-Gradient algorithm. Decoding the image sequences gives a set of high-level sequence of segments to execute the task in a given situation. We first show its application for a pick-and-place task with the Baxter robot while avoiding a moving obstacle from kinesthetic demonstrations, followed by a vision-based suturing task from surgical JIGSAWS dataset.
Bibtex reference
@article{Tanwani21, author="Tanwani, A. K. and Yan, A. and Lee, J. and Calinon, S. and Goldberg, K.", title="Sequential Robot Imitation Learning from Observations", journal="International Journal of Robotics Research ({IJRR})", year="2021", volume="40", number="10--11", pages="1306--1325" }