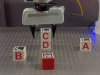
Zhang, Y., Xue, T., Razmjoo, A. and Calinon, S. (2024)
Logic Learning from Demonstrations for Multi-step Manipulation Tasks in Dynamic Environments
IEEE Robotics and Automation Letters (RA-L), 9:8, 7214-7221.
Abstract
Learning from Demonstration (LfD) stands as an efficient framework for imparting human-like skills to robots. Nevertheless, designing an LfD framework capable of seamlessly imitating, generalizing, and reacting to disturbances for long-horizon manipulation tasks in dynamic environments remains a challenge. To tackle this challenge, we present Logic-LfD, which combines Task and Motion Planning (TAMP) with an optimal control formulation of Dynamic Movement Primitives (DMP), allowing us to incorporate motion-level via-point specifications and to handle task-level variations or disturbances in dynamic environments. We conduct a comparative analysis of our proposed approach against several baselines, evaluating its generalization ability and reactivity across three long-horizon manipulation tasks. Our experiment demonstrates the fast generalization and reactivity of Logic-LfD for handling task-level variants and disturbances in long-horizon manipulation tasks.
Bibtex reference
@article{Zhang24RAL, author={Zhang, Y. and Xue, T. and Razmjoo, A. and Calinon, S.}, title={Logic Learning from Demonstrations for Multi-step Manipulation Tasks in Dynamic Environments}, journal={{IEEE} Robotics and Automation Letters ({RA-L})}, year={2024}, volume={9}, number={8}, pages={7214--7221}, doi={10.1109/LRA.2024.3418276} }