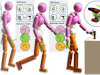
Liu, J., Li, Z., Yu, M., Dong, Z., Calinon, S., Caldwell, D.G. and Chen, F. (2025)
Human-Humanoid Robots Cross-Embodiment Behavior-Skill Transfer Using Decomposed Adversarial Learning from Demonstration
IEEE Robotics and Automation Magazine (RAM).
Abstract
Humanoid robots are envisioned as embodied intelligent entities capable of performing diverse human-level loco-manipulation tasks. However, effectively transferring human embodiment manipulation skills to humanoid robots poses significant challenges due to their differing configurations, dynamics, and coordination requirements across their high degree of freedom. In this work, we propose an efficient and transferable framework for transferring human whole-body loco-manipulation skills to various heterogeneous humanoid robots. We introduce a unified digital human model as a prototype embodiment to learn behavior primitives through adversarial imitation using human demonstrations. The high degree of freedom in humanoid robots is decomposed into multiple functional parts, where behavior primitives are learned separately and then coordinated. Loco-manipulation skills with generalized task parameters are achieved by dynamically combining these primitives using a human-object interaction graph as guidance. For each humanoid robot, the same loco-manipulation can be learned via embodiment-specific kinematic motion retargeting and dynamic fine-tuning.
Bibtex reference
@article{Liu25RAM, author={Liu, J. and Li, Z. and Yu, M. and Dong, Z. and Calinon, S. and Caldwell, D. G. and Chen, F.}, title={Human-Humanoid Robots Cross-Embodiment Behavior-Skill Transfer Using Decomposed Adversarial Learning from Demonstration}, journal={{IEEE} Robotics and Automation Magazine ({RAM})}, year={2025}, }