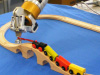
Calinon, S., Pistillo, A. and Caldwell, D.G. (2011)
Encoding the time and space constraints of a task in explicit-duration Hidden Markov Model
In Proc. of the IEEE/RSJ Intl Conference on Intelligent Robots and Systems (IROS), San Francisco, CA, USA, pp. 3413-3418.
Abstract
We study the use of different weighting mechanisms in robot learning to represent a movement as a combination of linear systems. Kinesthetic teaching is used to acquire a skill from demonstrations which is then reproduced by the robot. The behaviors of the systems are analyzed when the robot faces perturbation introduced by the user physically interacting with the robot to momentarily stop the task. We propose the use of a Hidden Semi-Markov Model (HSMM) representation to encapsulate duration and position information in a robust manner with parameterization on the involvement of time and space constraints. The approach is tested in simulation and in two robot experiments, where a 7 DOFs manipulator is taught to play a melody by pressing three big keys and to pull a model train on its track.
Bibtex reference
@inproceedings{Calinon11IROS, author="Calinon, S. and Pistillo, A. and Caldwell, D. G.", title="Encoding the time and space constraints of a task in explicit-duration hidden {M}arkov model", booktitle="Proc. {IEEE/RSJ} Intl Conf. on Intelligent Robots and Systems ({IROS})", year="2011", month="September", address="San Francisco, CA, USA", pages="3413--3418" }
Video
A Barrett WAM 7 DOFs manipulator learns to play a melody by pressing three big keys and learns to pull a model train on its track.
Kinesthetic teaching is used to acquire the skills from demonstrations. During reproduction, the robot faces perturbation introduced by the user physically interacting with the robot to momentarily stop the task.
We propose the use of a Hidden Semi-Markov Model (HSMM) representation to encapsulate duration and position information in a robust manner with parameterization on the involvement of time and space constraints.
Without perturbation, both types of parameterization produce similar behavior. By temporary holding the robot hand, biasing the system to one type of constraints (temporal or spatial weight) produce different behaviors, by continuing the progress of the weights in the melody playing task, and by freezing the current weights in the model railway task. In the melody playing task, the path followed by the robot takes a shortcut to recover from the time delay induced by the perturbation. In the model railway task, the robot continues the motion without changing the shape of the path.
Source codes
Download
Download Continuous HSMM sourcecode
Usage
Unzip the file and run 'demo1' in Matlab.
Reference
- Calinon, S., Pistillo, A. and Caldwell, D.G. (2011) Encoding the time and space constraints of a task in explicit-duration hidden Markov model. Proc. of the IEEE/RSJ Intl Conf. on Intelligent Robots and Systems (IROS).
Demo 1 - Simple example of continuous HSMM
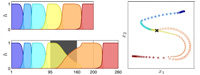
Simple example of the use of Hidden Semi-Markov Model (HSMM), a form of explicit-duration Hidden Markov model, to learn and reproduce trajectories.
The movement is represented as a combination of linear systems with a velocity dx computed iteratively as dx = sum_i h_i (A_i x + b_i), where h_i is a weight defined by HSMM.
A_i and b_i form a matrix and vector associated with state i of the HSMM.