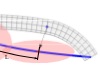
Bruno, D., Calinon, S. and Caldwell, D.G. (2014)
Null space redundancy learning for a flexible surgical robot
In Proc. of the IEEE Intl Conf. on Robotics and Automation (ICRA), Hong Kong, China, pp. 2443-2448.
Abstract
A new challenge for surgical robotics is placed in the use of flexible manipulators, to perform procedures that are impossible for currently available rigid robots. Since the surgeon only controls the end-effector of the manipulator, new control strategies need to be developed to correctly move its flexible body without damaging the surrounding environment. This paper shows how a positional controller for a new surgical robot (STIFF-FLOP) can be learnt from the demonstrations given by an expert user. The proposed algorithm exploits the variability of the task to comply with the constraints only when needed, by implementing a minimal intervention principle control strategy. The results are applied to scenarios involving movements inside a constrained environment and disturbance rejection.
Bibtex reference
@inproceedings{Bruno14ICRA, author="Bruno, D. and Calinon, S. and Caldwell, D. G.", title="Null Space Redundancy Learning for a Flexible Surgical Robot", booktitle="Proc. {IEEE} Intl Conf. on Robotics and Automation ({ICRA})", year="2014", month="May-June", address="Hong Kong, China", pages="2443--2448" }
Video
In minimally invasive surgery, tools go through narrow openings and manipulate soft organs to perform surgical tasks. There are limitations to current robot-assisted surgical systems due to the rigidity of robot tools. The aim of the STIFF-FLOP European project is to develop a soft robotic arm to perform surgical tasks by actively controlling the selected body parts of the robot. The flexibility of the robot allows the surgeon to move within organs to reach remote areas of the body and perform challenging procedures in laparoscopy.
The surgeon controls the end-effector during the surgical task, leaving the motion of the whole arm to the control and learning modules. The latter should drive the body of the robot along the trajectory followed by the surgeon, without applying pressure to or damaging the internal organs of the patients. The proposed learning algorithm works in the null space of the surgical manipulator, to avoid interfering with the surgeon and exploiting redundancy in an optimal way.