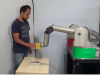
Rozo, L., Bruno, D., Calinon, S. and Caldwell, D.G. (2015)
Learning Optimal Controllers in Human-robot Cooperative Transportation Tasks with Position and Force Constraints
In Proc. IEEE/RSJ Intl Conf. on Intelligent Robots and Systems (IROS), Hamburg, Germany, pp. 1024-1030.
Abstract
Human-robot collaboration seeks to have humans and robots closely interacting in everyday situations. For some tasks, physical contact between the user and the robot may occur, originating significant challenges at safety, cognition, perception and control levels, among others. This paper focuses on robot motion adaptation to parameters of a collaborative task, extraction of the desired robot behavior, and variable impedance control for human-safe interaction. We propose to teach a robot cooperative behaviors from demonstrations, which are probabilistically encoded by a task-parametrized formulation of a Gaussian mixture model. Such encoding is later used for specifying both the desired state of the robot, and an optimal feedback control law that exploits the variability in position, velocity and force spaces observed during the demonstrations. The whole framework allows the robot to modify its movements as a function of parameters of the task, while showing different impedance behaviors. Tests were successfully carried out in a scenario where a 7 DOF backdrivable manipulator learns to cooperate with a human to transport an object.
Bibtex reference
@inproceedings{Rozo15IROS, author="Rozo, L. and Bruno, D. and Calinon, S. and Caldwell, D. G.", title="Learning Optimal Controllers in Human-robot Cooperative Transportation Tasks with Position and Force Constraints", booktitle="Proc. {IEEE/RSJ} Intl Conf. on Intelligent Robots and Systems ({IROS})", year="2015", month="Sept.-Oct.", address="Hamburg, Germany", pages="1024--1030" }
Video
This video shows how a robot can learn collaborative behaviors from human demonstrations. The collaborative behavior considers robot motion adaptation to parameters of a task, extraction of the desired robot state, and variable impedance control for human-safe interaction. The human demonstrations are probabilistically encoded by a task-parametrized formulation of a Gaussian mixture model. Such encoding is later used for specifying both the desired state of the robot, and an optimal feedback control law that exploits the variability in position, velocity and force spaces observed during the demonstrations. The whole framework allows the robot to modify its movements as a function of parameters of the task, while showing different impedance behaviors.