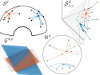
Calinon, S. (2020)
Gaussians on Riemannian Manifolds: Applications for Robot Learning and Adaptive Control
IEEE Robotics and Automation Magazine (RAM), 27:2, 33-45.
Abstract
This article presents an overview of robot learning and adaptive control applications that can benefit from a joint use of Riemannian geometry and probabilistic representations. The roles of Riemannian manifolds, geodesics and parallel transport in robotics are first discussed. Several forms of manifolds already employed in robotics are then presented, by also listing manifolds that have been underexploited but that have potentials in future robot learning applications. A varied range of techniques employing Gaussian distributions on Riemannian manifolds is then introduced, including clustering, regression, information fusion, planning and control problems. Two examples of applications are presented, involving the control of a prosthetic hand from surface electromyography (sEMG) data, and the teleoperation of a bimanual underwater robot. Further perspectives are finally discussed, with suggestions of promising research directions.
Bibtex reference
@article{Calinon20RAM, author="Calinon, S.", title="Gaussians on {R}iemannian Manifolds: Applications for Robot Learning and Adaptive Control", journal="{IEEE} Robotics and Automation Magazine ({RAM})", year="2020", month="June", volume="27", number="2", pages="33--45", doi="10.1109/MRA.2020.2980548" }
Video
Related publication: Calinon, S. (2020). Gaussians on Riemannian Manifolds: Applications for Robot Learning and Adaptive Control. IEEE Robotics and Automation Magazine (RAM), 27:2, 33-45.
Source codes
Source codes related to this publication are available as part of PbDlib.