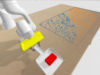
Kulak, T. and Calinon, S. (2023)
Combining Social and Intrinsically-Motivated Learning for Multi-Task Robot Skill Acquisition
IEEE Trans. on Cognitive and Developmental Systems, 15:2, 385-394.
Abstract
This article proposes an approach for coupling internally-guided learning and social interaction in the context of a multi-task robot skill acquisition framework. More specifically, we focus on learning a parametrized distribution of robot movement primitives by combining active intrinsically-motivated learning and active imitation learning. We focus on the case where the learning modalities to use are not specified in advance by the experimenter, but are chosen actively by the robot through experiences. Such approach aims at combining experiential and observational learning as efficiently as possible, by relying on a skill acquisition mechanism in which the agent/robot can orchestrate different learning strategies in an iterative manner, and modulate the use of these modalities based on previous experiences. We demonstrate the effectiveness of our approach on a waste throwing task with a simulated 7-DoF Franka Emika robot, where at each iteration of the learning process the robot can actively choose between observational/imitation learning and experiential/intrinsically-motivated learning.
Bibtex reference
@article{Kulak23TCDS, author="Kulak, T. and Calinon, S.", title="Combining Social and Intrinsically-Motivated Learning for Multi-Task Robot Skill Acquisition", journal="{IEEE} Trans. on Cognitive and Developmental Systems", year="2023", volume="15", number="2", pages="385--394", doi="10.1109/TCDS.2021.3069341" }