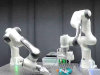
Jankowski, J., Racca, M. and Calinon, S. (2022)
From Key Positions to Optimal Basis Functions for Probabilistic Adaptive Control
IEEE Robotics and Automation Letters (RA-L), 7:2, 3242-3249.
Abstract
In the field of Learning from Demonstration (LfD), movement primitives learned from full trajectories provide mechanisms to generalize a demonstrated skill to unseen situations. Key position demonstrations, requiring the user to provide only a sequence of via-points rather than a complete trajectory, have been shown to be an appealing alternative. In this letter, we investigate the synergy between learning adaptive movement primitives and key position demonstrations. We exploit a linear optimal control formulation to (1) recover the timing information of the skill missing from key position demonstrations, and to (2) infer low-effort movements on-the-fly. We evaluate the performance of the proposed approach in a user study where 16 novice users taught a 7-DoF robot manipulator, showing improved learning efficiency and trajectory smoothness. We further showcase the effectiveness of the approach for tasks that require precise demonstrations and on-the-fly movement adaptation.
Bibtex reference
@article{Jankowski22RAL, author="Jankowski, J. and Racca, M. and Calinon, S.", title="From Key Positions to Optimal Basis Functions for Probabilistic Adaptive Control", year="2022", journal="{IEEE} Robotics and Automation Letters ({RA-L})", volume="7", number="2", pages="3242--3249", doi="10.1109/LRA.2022.3146614" }